Deep Learning
Technology Overview
Deep learning, which is a subset of artificial intelligence (AI), is based on models trained with large amounts of data and can perform extensive processing tasks, such as image and voice recognition and natural language processing, under specific conditions.
This is a type of machine learning, specifically a method that uses multilayer neural networks to automatically extract and learn characteristics from data. A neural network is a model that mimics the function of neurons in the human brain and generally consists of an input layer, a data processing layer, and an output layer, designed to enable complex processing.
Click to enlarge
Deep learning extracts various features from image data obtained from cameras and microscopes, enabling tasks such as object recognition and segmentation to provide the information users want. It also requires real-time processing for tasks like object recognition in cameras and cell tracking. In industrial applications, it removes noise from X-ray CT images to reveal minute defects in parts.
Technology Application Examples
Total imaging solution NIS-Elements
The NIS-Elements imaging software for microscopes is equipped with image processing functions using deep learning technology. By generating clear images from unclear images acquired at low excitation light intensity to avoid phototoxicity in fluorescence observation, it enables accurate and detailed dynamic analysis of cells and other subjects. In addition, the image processing function that removes blurred light from outside the focal plane allows you to accurately capture the structure of the target object.
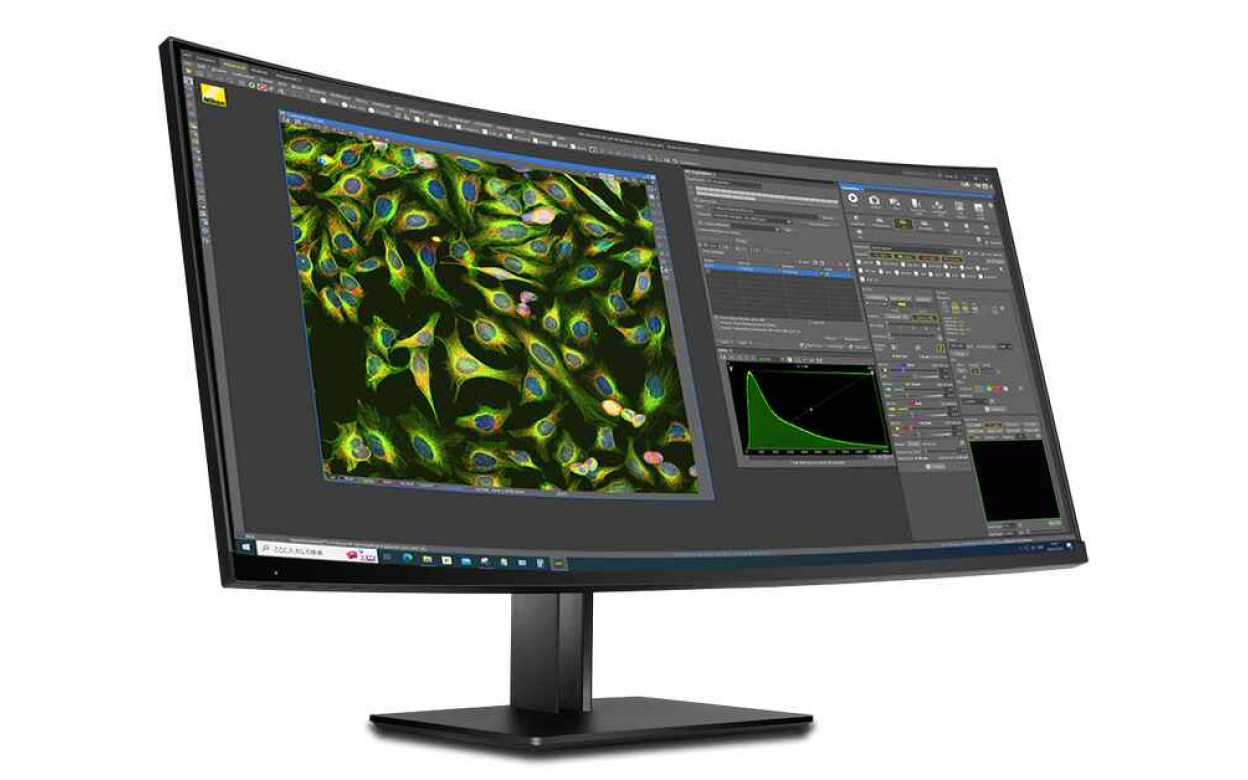
Technologies related to these examples
Related Technology
Data Science
Methods from various academic fields, such as statistics and information engineering, can extract useful knowledge from data. With the spread of data communication infrastructure, like the Internet, a wide range of information is now stored as data. Using this large amount of data, it becomes possible to make judgments and predictions in systems involving complex events.
Data science is necessary not only for image recognition using machine learning, but also for accumulating and analyzing appropriate data, building and verifying models, and detecting and predicting abnormalities for the stable operation of lithography systems. In order to appropriately collect data related to the desired insights, a wide range of knowledge is required, including understanding the mechanisms of target equipment and instruments, as well as methods for both data analysis and model construction. It is also important to provide feedback on how to obtain data that can be easily processed by machines rather than people.
Main Related Products
You can search for articles related to Nikon’s technology, research and development by tag.