Nikon releases NIS-A NIS.ai, an Artificial Intelligence (AI) based module for microscopes as a workflow improvement solution
January 22, 2020
TOKYO — Nikon Corporation (Nikon) is pleased to announce the release of NIS-A NIS.ai (NIS.ai), an AI module for microscopes that enables advanced image processing and analysis using artificial intelligence (AI).
NIS.ai is a dedicated module for NIS-Elements, Nikon's imaging software that integrates acquisition, analysis, and data management of microscopic images. The module utilizes convolutional neural networks to learn from small training datasets supplied by the user. The training results can then be easily applied to process and analyze large volumes of data, increasing throughput and expanding application limits. NIS.ai has three functions: Enhance.ai for obtaining low-noise images with short exposure times, Convert.ai for generating virtual fluorescent images from unstained samples, and Segment.ai for easy segmentation of complex cells and structures.
Research in the medical and biological sciences has become deeper and more diversified. There is a growing need for detailed high-speed imaging of live, preferably un-stained, biological samples and efficient analysis of massive amounts of data. In addition, researchers are constantly looking for ways to reduce phototoxic effects of imaging and to preserve viability of the samples. Nikon endeavors to support researchers by enhancing imaging workflows, reducing the need for manual input and associated time and cost, as well as expanding application possibilities through AI-based solutions.
Product Overview
Swipe horizontally to view full table.
Name of Product | AI module for microscopes ”NIS-A NIS.ai“ |
---|---|
Release Date | January 2020 (Dependent on region) |
Major Features
1. Enhance.ai produces low-noise microscopic images with short exposure times
Enhance.ai restores details to under-exposed, noisy images, resulting in clear images with high signal-to-noise ratio. Enhance.ai allows users to acquire images with short exposure times without sacrificing signal-to-noise, thus expanding practical applications for low-light imaging. For example, using short exposure times reduces phototoxicity and photobleaching leading to improved cell viability and enabling longer or more frequent time-lapse image acquisitions.
Image Example
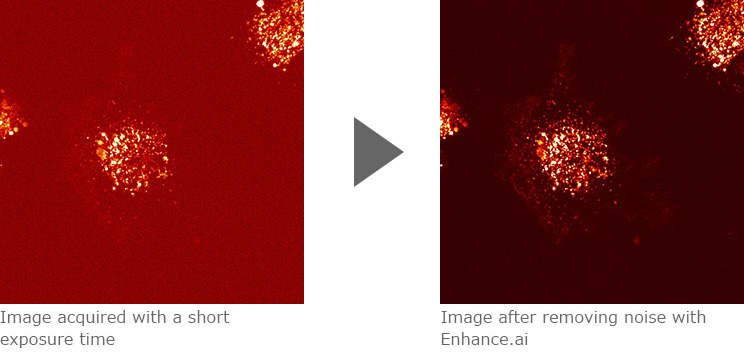
Confocal images of CD63 (membrane protein) labeled with mCherry (fluorescence protein) expressed in HT1080 cells (human fibrosarcoma). Enhance.ai can be used to improve noisy fluorescence images acquired with a short exposure time of 10 msec. After processing with Enhance.ai, the intracellular distribution of the labeled membrane protein is clearly visible.
Images courtesy of Dr. Yoshitaka Shirasaki, Graduate School of Science, The University of Tokyo
Sample courtesy of Dr. Kiyotaka Shiba, Japanese Foundation for Cancer Research
2. Convert.ai generates images resembling fluorescence images from unstained cell images
Based on training datasets, Convert.ai can predict and produce virtual fluorescent images from differential interference-contrast or phase-contrast images of unstained specimen. Virtual images generated by Convert.ai can be used for image segmentation and downstream analysis, freeing up imaging channels and eliminating the need to fix and stain samples for certain applications. Virtual fluorescent images also enable non-destructive, in-line analysis of unstained, live samples, expanding application possibilities.
Image Example
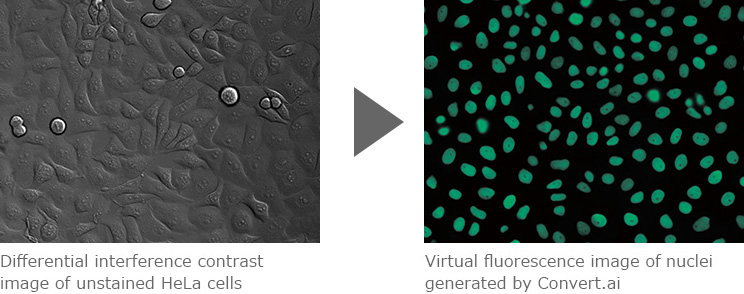
Convert.ai can be trained to predict where the DAPI label would be based on unstained DIC (or phase-contrast) images. This enables users to perform nuclei-based image analysis without having to stain samples with DAPI or acquire a fluorescent channel.
Images courtesy of Dr. Kentaro Kobayashi, Division of Technical Staff, Research Institute for Electronic Science, Hokkaido University
3. Segment.ai enables easy extraction of target cells and structures
Structures and cell types that are difficult to define by classic thresholding can be easily identified and segmented using Segment.ai. For example, Segment.ai can be used to easily extract a specific cell type from differential interference or phase contrast images containing a mixture of cell types. Conventional segmentation methods require manual input and significant time and effort. Segment.ai can be trained on a small subset of manually segmented datasets to quickly and automatically detect and segment structures from thousands of datasets. This tool reduces the amount of time needed to carry out segmentation-based analysis as well as reducing human-induced bias.
Image Example
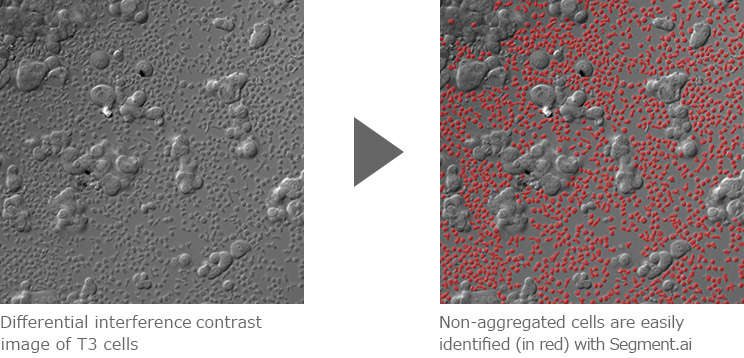
Segment.ai was used to identify and segment non-aggregated cells from an image containing a mixture of aggregated and non-aggregated cells, acquired by differential interference-contrast microscopy. Segmented images can then be used for downstream analysis including measurement, counting and tracking.
Images courtesy of: Dr. Simon C. Watkins, Department of Cell Biology, University of Pittsburgh
The information is current as of the date of publication. It is subject to change without notice.